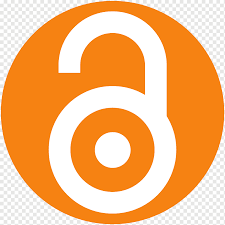
DYNAMIC PLANT INSIGHTS: REAL-TIME MONITORING OF PHOTOSYNTHETIC DYNAMICS
Baskoro Sinaga , Department of Informatics Engineering, Ma Chung Research Centre for Photosynthetic Pigments, Universitas Ma Chung, Malang, IndonesiaAbstract
This study introduces a novel approach for the real-time monitoring of plant photosynthetic pigments to enable dynamic assessment of plant health and physiological responses. By employing cutting-edge sensing technologies and data analysis techniques, our method offers continuous and non-invasive measurement of chlorophyll, carotenoids, and other key pigments crucial for photosynthesis. We demonstrate the applicability of our approach across various plant species and environmental conditions, highlighting its potential for enhancing our understanding of plant physiology, optimizing agricultural practices, and assessing ecosystem health.
Keywords
Plant physiology, Photosynthetic pigments, Real-time monitoring
References
Anggraini T. 2017. Antioxidant activity of Syzygium oleana.Pakistan J. Nutr. 16:605-11.
Croft H., Chen J.M. 2017. Leaf pigment content, comprehensiveremote sensing. Elsevier Inc., Amsterdam, Netherlands.
Cruz A., Ampatzidis Y., Pierro R., Materazzi A., Panattoni A., DeBellis L., Luvisi A. 2019. Detection of grapevine yellowssymptoms in Vitis vinifera L. with artificial intelligence.Comput. Electron. Agric. 157:63-76.
Esteban R., Barrutia O., Artetxe U., Fernández-Marín B.,Hernández A., García-Plazaola J.I. 2015. Internal and externalfactors affecting photosynthetic pigment composition inplants: a meta-analytical approach. New Phytol. 206:268-80.
Gallego P.P., Gago J., Landín M. 2011. Artificial neural networkstechnology to model and predict plant biology process. In: K.Suzuki (ed.) Artificial neural networks - methodologicaladvances and biomedical applications. InTech., London, UK,pp 197-216.
Ghazi M.M., Yanikoglu B., Aptoula E. 2017. Plant identificationusing deep neural networks via optimization of transfer learn-ing parameters. Neurocomputing 235:228-35.
Gitelson A.A., Merzlyak M.N. 2004. Non-destructive assessmentof chlorophyll, carotenoid and anthocyanin content in higherplant leaves: principles and algorithm. In: S. Stamatiadis, J.M.Lynch, J.S. Schepers (Ed.), Remote sensing for agriculture andthe environment. Greece Ella, pp 78-94.
Gitelson A.A., Merzlyak M.N., Chivkunova O.B. 2001. Opticalproperties and nondestructive estimation of anthocyanin con-tent in plant leaves. Photochem. Photobiol. 74:38-45.
Gitelson A., Solovchenko A. 2017. Generic algorithm for estimat-ing foliar pigment content. Geophys. Res. Lett. 44:9293-98.
Gitelson A., Solovchenko A. 2018. Non-invasive quantification offoliar pigments: possibilities and limitations of reflectance andabsorbance-based approaches. J. Photochem. Photobiol. BBiol. 17:537-44.
Huang W.D., Lin K.H., Hsu M.H., Huang M.Y., Yang Z.W., ChaoP., Yang C.M. 2014. Eliminating interference by anthocyaninin chlorophyll estimation of sweet potato (Ipomoea batatas L.)leaves. Bot. Stud. 55:1-10.
Inácio D., Rieder R. 2018. Computer vision and artificial intelli-gence in precision agriculture for grain crops : a systematicreview. Comput. Electron. Agric. 153:69-81.
Article Statistics
Copyright License
Copyright (c) 2024 Baskoro Sinaga

This work is licensed under a Creative Commons Attribution 4.0 International License.