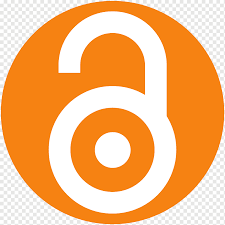
ARTIFICIAL NEURAL NETWORKS FOR ENHANCED UAV PERFORMANCE IN URBAN AREA INSPECTIONS
Paulo Carvalho , Institute of Mathematics and Computation-IMC, Federal University of Itajuba, BrazilAbstract
Urban area inspections often present unique challenges for unmanned aerial vehicles (UAVs), requiring adaptable and responsive systems to navigate complex, dynamic environments. This study explores the integration of Artificial Neural Networks (ANN) to enhance the capabilities of small UAVs specifically for urban inspections. By leveraging ANN models, UAVs can improve obstacle avoidance, optimize flight paths, and enhance image processing for real-time data analysis, all of which are critical in densely populated and infrastructure-heavy areas. We conducted a case study to evaluate the performance of ANN-enabled UAVs in typical urban scenarios, assessing improvements in operational efficiency, safety, and accuracy. The findings suggest that incorporating ANN significantly enhances UAV performance, offering a robust solution for detailed urban inspections, monitoring, and data acquisition. This research contributes to the development of autonomous UAV systems capable of addressing the demands of urban environments with high reliability and minimal human intervention.
Keywords
Artificial Neural Networks (ANN), Unmanned Aerial Vehicles (UAVs), Urban Area Inspection
References
Wang Y, Schultz RR, Fevig RA. Sensor fusion method using. GPS/IMU data for fastUAV surveillance video frame registration. IEEE International Conference onAcoustics, speech and signal processing–ICASSP. 2009;985-988.
Semsch E, Jakob M, Pavlíćek D, Pěchouček M. Autonomous UAV surveillance incomplex urban environments. International Conference on Web Intelligent AgentTechnology Workshos. IEEE/WIC/ACM. 2009;82-85.
Mejias LO, Saripalli S, Couvera P, Sukhatme CS.Visual servoing of an autonomoushelicopterin urban areas using feature tracking. Journal of Field Robotics.2006;23(3):185-199.
Hrabar S, Merz T, Frousheger D. Development of an autonomous helicopter for aerialpowerline inspections. 2010 1st International Conference on Applied Robotics forthePower Industry (CARPI 2010).2010;1–6.
Haykin S. Self-organizing maps. Neural networks-A comprehensive foundation, 2nded. Prentice-Hall; 1999.
Paparazzi Project Webpage; 2012.[Online]. Available at Internet:http://paparazzi.enac.fr/wiki/Main_Page. [Accessed: 15-Jun-2012].
Paparazzi Project Webpage, Ground Control Station; 2012. [Online]. Available atInternet:http://paparazzi.enac.fr/wiki/GCS. [Accessed: 12-May-2012].
Gonzales RC, Woods ER. Processamentode Imagens Digitais. São Paulo, Brasil:Editora Edgard Blücher; 2000.
Machado A. Neuroanatomia Funcional. Livraria Atheneu; 1980.
Rowsan Jahan Bhuiyan, Salma Akter, Aftab Uddin, Md Shujan Shak, Md Rasibul Islam, S M Shadul Islam Rishad, Farzana Sultana, & Md. Hasan-Or-Rashid. (2024). SENTIMENT ANALYSIS OF CUSTOMER FEEDBACK IN THE BANKING SECTOR: A COMPARATIVE STUDY OF MACHINE LEARNING MODELS. The American Journal of Engineering and Technology, 6(10), 54–66. https://doi.org/10.37547/tajet/Volume06Issue10-07
Md Habibur Rahman, Ashim Chandra Das, Md Shujan Shak, Md Kafil Uddin, Md Imdadul Alam, Nafis Anjum, Md Nad Vi Al Bony, & Murshida Alam. (2024). TRANSFORMING CUSTOMER RETENTION IN FINTECH INDUSTRY THROUGH PREDICTIVE ANALYTICS AND MACHINE LEARNING. The American Journal of Engineering and Technology, 6(10), 150–163. https://doi.org/10.37547/tajet/Volume06Issue10-17
Md Salim Chowdhury, Md Shujan Shak, Suniti Devi, Md Rashel Miah, Abdullah Al Mamun, Estak Ahmed, Sk Abu Sheleh Hera, Fuad Mahmud, & MD Shahin Alam Mozumder. (2024). Optimizing E-Commerce Pricing Strategies: A Comparative Analysis of Machine Learning Models for Predicting Customer Satisfaction. The American Journal of Engineering and Technology, 6(09), 6–17. https://doi.org/10.37547/tajet/Volume06Issue09-02
Md Shujan Shak, Md Shahin Alam Mozumder, Md Amit Hasan, Ashim Chandra Das, Md Rashel Miah, Salma Akter, & Md Nur Hossain. (2024). OPTIMIZING RETAIL DEMAND FORECASTING: A PERFORMANCE EVALUATION OF MACHINE LEARNING MODELS INCLUDING LSTM AND GRADIENT BOOSTING. The American Journal of Engineering and Technology, 6(09), 67–80. https://doi.org/10.37547/tajet/Volume06Issue09-09
Md Abu Sayed, Badruddowza, Md Shohail Uddin Sarker, Abdullah Al Mamun, Norun Nabi, Fuad Mahmud, Md Khorshed Alam, Md Tarek Hasan, Md Rashed Buiya, & Mashaeikh Zaman Md. Eftakhar Choudhury. (2024). COMPARATIVE ANALYSIS OF MACHINE LEARNING ALGORITHMS FOR PREDICTING CYBERSECURITY ATTACK SUCCESS: A PERFORMANCE EVALUATION. The American Journal of Engineering and Technology, 6(09), 81–91. https://doi.org/10.37547/tajet/Volume06Issue09-10
Article Statistics
Copyright License
Copyright (c) 2024 Paulo Carvalho

This work is licensed under a Creative Commons Attribution 4.0 International License.